Towards Building Multicultural and Multilingual Safe Large Language Models
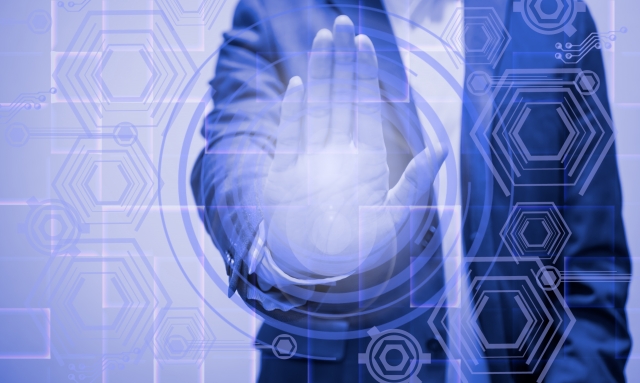
Download the report on the webinar here.
Download the report on the Singapore AI Safety Red Teaming Challenge here.
Date(s) | Monday, 11 November 2024, 10:00-11:00 JST |
---|---|
Venue |
Zoom Webinar (Register here) |
Registration | Pre-registration required (If you cannot attend on the day but would like to be informed of the youtube link at a later date, please register) |
Language | Japanese |
Abstract |
As generative AI becomes more widely used, it is crucial for AI models to accurately reflect cultural and linguistic risks in different regions. Identifying harmful content specific to each culture must be continuously updated. This requires collaboration between AI researchers, social scientists, policymakers, and practitioners to form a global community for ongoing discussions.
One approach, known as “red teaming,” involves intentionally trying to break AI models by inducing harmful content, but current red teaming efforts are mostly Western-focused.
To address this gap, Singapore’s Infocomm Media Development Authority launched a Singapore AI Safety Red Teaming Challenge in November 2024, gathering experts in culture and language to enhance AI safety in specific regions. Japan is also involved in this initiative. This event will report on the project’s November meeting and discuss frameworks to sustain such communities, welcoming those interested in AI safety and governance. |
Program |
10:00-10:15 Opening Remarks and Overview: Arisa EMA (Tokyo College, The University of Tokyo)
10:15-10:30 Efforts Toward LLM Safety: Satoshi SEKINE (NII-LLMC/RIKEN AIP)
10:30-10:45 Overview of the CTF Challenge and Future Plans: Teresa TSUKIJI (Japan Deep Learning Association)
10:45-11:00 Comments and Q&A |
Contact | tg-event@tc.u-tokyo.ac.jp |
Organized by | Tokyo College, The University of Tokyo; Institute for Future Initiatives, The University of Tokyo; Next Generation Artificial Intelligence Research Center, The University of Tokyo; B'AI Global Forum, The University of Tokyo; Japan Deep Learning Association |
Supported by | National Institute of Informatics, Research and Development Center for Large Language Models; Japan AI Safety Institute |